ai
Daring Fireball: Siri Is Super Dumb and Getting Dumber
Daring Fireball: Siri Is Super Dumb and Getting Dumber:
Old Siri — which is to say pre-Apple-Intelligence Siri — does OK on this same question. On my Mac running MacOS 15.1.1, where ChatGPT integration is not yet available, Siri declined to answer the question itself and provided a list of links, search-engine-style, and the top link was to this two-page PDF listing the complete history of North Dakota’s Class A boys’ and girls’ champions, but only through 2019. Not great, but good enough.
New Siri — powered by Apple Intelligence™ with ChatGPT integration enabled — gets the answer completely but plausibly wrong, which is the worst way to get it wrong. It’s also inconsistently wrong — I tried the same question four times, and got a different answer, all of them wrong, each time. It’s a complete failure.
I’ve been saying for a while that most LLM-based “assistants” will be worse than what they replace. Older assistants were limited in domain, but largely pretty good at what they could do. LLM-powered assistants don’t know their own limitations.
Ten Blue Links "my god, what have I done?" edition
1. Well who could possibly have seen this coming?
I wrote a while ago that the era of major levels of affiliate revenue for publishers was going to come to an end within the next three to five years. Generative AI writing means both that Google is likely to become a sea of slop, and that anyone with a search engine – especially Google – is going to cream off the best quality search results for itself.Amazon is taking this a step further by using generative AI to do product recommendations on site. Given that a large number of searches for products begin on Amazon anyway, this is more bad news for anyone who makes money from sending traffic towards the Seattle company. And as users get more and more exposed to using conversation to hone down what they want, this is going to get worse for publishers who focus on “an article” as the canonical way of recommending products.
The truth is that articles have never been brilliant at recommending the right solution for any individual. For example, the answer to “what car is right for me” has always depended on your use of it. Conversational agents using good quality data will be a better solution in the long run.
2. Turkeys, meet Christmas
Yes, I know that advertising revenue is toast, but if you are a major publisher and you’re giving OpenAI the rights to mine your content, you are silly. The sum of money they’re paying is never going to go up: and when your licensing deal ends, they will have used everything you have ever done to train a model which can recreate your style of content in seconds. Golf, as they say, clap.3. Possible sign of the end times: I agree with DHH
David Heinemeier Hansson is not on my Christmas card list. He’s one of those techbros for whom the phrase “arrogant asshat” is entirely appropriate. But for once, I’m going to agree wholeheartedly with something he wrote: Automattic demanding a tithe from WP Engine is a violation of the ideals of open source software, reduces trust in it, and in my view shows that Matt Mullenweg’s “principles” begin and end at maintaining control over WordPress.4. Where all the Chief Metaverse Officers gone?
Good question. My bet is the B Ark.5. Oh boy, Roblox is toast
Where “toast” means “full of child grooming”. Ouch.6. Quote of the week
The truth is the news media is effectively in the tank for Trump, sanewashing his literal nonsense, outright lies, and violence-inspiring hate speech against even legal immigrants. But our major political news media remains so hyper-focused on appearing not to favor one political side over the other that it’s completely lost sight of what ought to be their north star: the truth. John Gruber, “Why Is Jack Smith’s Unsealed Motion, Outlining Trump’s Criminal Actions to Overturn the 2020 Election, Not the Top Story?”
7. Elon, phone home (from Mars)
I increasingly wonder why Elon Musk is bothering trying to establish himself on Mars, and not just because it looks like a complete dump up there. (Seriously, if you think that’s beautiful, I have around a hundred thousand disused quarries I’d love to show you right here on Earth.) The ever-wonderful Marina Hyde, wondering what reality Musk occupies.
8. I’m shocked, shocked I tell you that lovely Google would do this
Yeah no of course I’m not. Turns out that Google Pixel phones give it your location, email address and more every fifteen minutes, without consent. And no, before you say something, using an iPhone isn’t much a miracle cure.9. This stuff matters
I could have written a WordPress special edition this time out. But I wondered if that would be too “insider baseball” for most people.But a big chunk of the internet runs on WordPress. Publishers use it a lot. It’s become the IBM of web servers: “no one ever got fired for recommending WordPress”. And the hold-outs in the publishing space who have had their own bespoke software or used something else appear to be dwindling every year.
So WordPress matters, to a degree that few other software platforms do. It became popular in part because it was open source, so anyone could customize it and bend it to their will, and because so many people used it that it was easy to support and find developers for. It saw off semi-forgotten closed source rivals.
If you want a summary then Mathew Ingram’s article is a good place to go. Mathew has written something which encapsulates the feeling that I think many people have: profound disappointment in Mat Mullenweg’s behaviour, in his refusal to understand that being both the CEO of WordPress.com and the effective owner of WordPress.org places him in a position which needs to be handled sensitively. Using WordPress.org to attack a commercial rival of his company means it “now looks like the CEO of a multibillion-dollar corporation is using his control of a theoretically open-source foundation to extort money from a competitor.” That is unacceptable.
10. A hole is a hole
“There is no such thing as a magic hole that only good guys can use”. Wendy Grossman has spent a long time pointing out that if you build a backdoor in a system to let “good guys” in law enforcement use, you’re opening the same thing for people who you would really rather not let into systems. And so it goes.Ten Blue Links, literary salon Edition
1. Apple’s built in apps can do (almost) everything
One of the characteristics of hardcore nerdery is the tendency to over engineer your systems. People spend a lot of time creating systems, tinkering with them, making them as perfect as possible, only to abandon them a few years down the line when some new shiny hotness appears.I’m as guilty of this as the next nerd, but at least I’m aware of my addiction. It’s one of the reasons why I have spent time avoiding getting sucked into the word of Notion, because I can see myself losing days (weeks) to tinkering, all the while getting nothing done.
That said, if you are going to create an entire workflow management system and you’re in the word of Apple, you could do a lot worse than take a leaf out of Joan Westenberg’s book and use all Apple’s first party apps. They have now got to the point where they are superficially simple, but contain a lot of power underneath.
The downside is it’s an almost certain way of trapping yourself in Apple’s ecosystem for the rest of time. Yes, Apple’s services – which lie behind the apps – use standards and have the ability to export, but not all of them, and for how long?
It’s a trade off, and from my perspective not one that really works for me right now. But if it does for you, then it’s a good option (and better than Notion).
2. Juno removed from the App Store
AKA “why I do not like any company, no matter how well intentioned, to have a monopoly on software distribution for a platform.” Christian Selig created a YouTube player for the Apple Vision Pro. It doesn’t block ads or do anything which could be regarded as dubious. But Google claimed it was using its trademarks, and Apple removed it.Why is this problematic? Because it’s setting Apple up as a judge in a legal case. YouTube could, and should, have gone to a judge if it believed it had a legal case for trademark violation. That’s what judges are for. Instead, probably because it knew that it wouldn’t win a case like that, it went to Apple. Apple (rightly) doesn’t want to get involved in trademark disputes, so it shrugged and removed the app.
This extra-legal application of law is one of the most nefarious impacts of App Store monopolies. And if it continues to be allowed, it will only get worse.
3. The horrible descent of Matt Mullenweg
You will be aware of the conflict between WordPress — by which we mean Matt Mullenweg, because according to Matt he is WordPress — and WPEngine. I have many opinions on this which I will, at some point, get down to writing. The most important one is simple: if you make an open source product under the GPL, you don’t get to dictate to anyone how they use it and don’t get to attempt to punish them for not contributing “enough”. Heck, you don’t get to decide what “enough” looks like.The whole thing has brought out the worst in Mullenweg, as evidenced in his attacks on Kellie Peterson. Peterson, who is a former Automattic employee, offered to help anyone leaving WordPress find opportunities. Mullenweg decided this was attacking him, and claimed this was illegal. I don’t know about you, but when a multi-millionaire starts to throw around words like “tortuous interference” I pay attention.
As with many of that generation of California ideologists Mullenweg appears to have decided that he knows best, now and always. Yes, private equity companies that use open source projects and contribute nothing back are douchebags, but they’re douchebags who are doing something that the principles of open source explicitly allow them to do. Mullenweg’s apparent desire to be the emperor of WordPress is worrying.
4. OpenAI raises money, still isn’t a business
Ed Zitron wrote an excellent piece this week on the crazy valuation and funding round which OpenAI just closed, pointing out that (1) ChatGPT loses money on every customer, and (2) there is no way to use scale to change this: the company is going to keep losing money on every customer as models get more compute-hungry. Neither Moore’s Law nor the economies of scale which made cloud services of the past profitable are going to come riding to the rescue.I think Ed’s right — and it’s important to note, as Satya Nadella did, that LLMs are moving into the “commodity” stage — but one other thing to note is that many of the more simple things which people use LLMs for are being pushed from cloud to edge. Apple’s “Apple Intelligence” is one example of this, but Microsoft is also pushing a lot of the compute down to the device level in the ARM-based Copilot PCs.
This trend should alleviate some of the growth issues that OpenAI has, but it’s a double-edged sword because it makes it less likely that someone will need to use ChatGPT, and so even less likely to need to pay OpenAI.
5. Why I love Angela Carter
I think I first read Angela Carter during my degree, one of the few books that I bothered to read for my literature modules1. This piece includes possibly my favourite quote from her: “Okay, I write overblown, purple, self-indulgent prose. So fucking what?”And the point is: sometimes it’s fine not to be subtle. Sometimes it’s fine to be overblown. Sometimes the story demands it, like a steak needs to be juicy.
6. And speaking of writers I love
I can’t tell you enough to just go and read M John Harrison. Climbers is sometimes regarded as his best novel, and this essay on why it’s the best book written about 21st century male loneliness despite being written in 1989 captures a lot of it. I like the line from Robert Macfarlane’s introduction: “To Harrison, all life is alien”. Amen to that.7. No really this week is all lit, all the time
Olivia Laing is another writer that makes me salivate when I read her. Like Harrison and Carter, her prose is as good as her fiction, and her recent book The Garden Against Time – an account of restoring a garden to glory – is one of the best yet. If you need any further persuading, you should read this piece in the New Yorker.8. Down in Brighton? Like books?
Next weekend is the best-named literary festival in the world down in Brighton. The Coast is Queer includes loads of brilliant sessions including queer fantastical reimaginings, the incredible Julia Armfield on world building, Juno Dawson’s trans literary salon, and the unmissable David Hoyle. I’m going, you should go.9. Harlan the terrible
Like Cory Doctorow, I grew up worshipping Harlan Ellison. And like Cory, as I’ve grown older I have see that Harlan was an incredibly complicated person. Cory has written a great piece (masquerading as just one part of a linkblog) which not only looks at Harlan, warts and all, but also talks about the genesis of the story he contributed to the – finally finished! – Last Dangerous Visions.10. Argh Mozilla wai u doo this?
No Mozilla, no, online advertising does not need “improving… through product and infrastructure”. Online advertising needs to understand that surveillance-based ads were always toxic and the whole thing needs to be torn up. I agree with Jamie Zawinski: Mozilla should be “building THE reference implementation web browser, and being a jugular-snapping attack dog on standards committees.”To be clear: I think Mozilla’s goals are laudable, in the sense that at the moment the choice for people is either accept being tracked to a horrendous degree or just block almost every ad and tracker. But you can’t engineer your way around the advertising industry’s rapacious desire for data. It’s that industry which needs to change, not the technology.
- I read a lot, I just didn’t read a lot that was actually on the syllabus. ↩︎
Ten Blue Links, AI is bad now edition
First up, apologies that there's been no long form post this week. I've had some family stuff which had to take priority over writing. Normal service should be resumed from next week.
And now on to the good stuff…
1. The last refuge of the desperate media
Ahh, low rent native ads — the kind that are designed to fool people into clicking by appearing to be genuine user or editorial posts. Always a sign that a company is desperate for revenue, any kind of revenue, and never mind the longer-term implications on quality. Now, why would Reddit want to do that?
2. Repeat after me: AI is not a thing
More specifically, AI is not a single technology, and what we talk about in the media as “AI” is, in fact, quite a limited, relatively new tool coming out of AI research — the Large Language Model, or LLM. Why does this matter? Because (how shall I put this?) less technically educated executives are likely to read articles like this one, about the successful use of AI in the oil industry, and think that they need to jump on the AI bandwagon by adopting LLMs. These are two very different things: Robowell, for example, is a machine learning system designed to automate specific tasks. It learns to do better as it goes along — something that LLMs don't do.
3. Tesla bubble go pop
The notion that Tesla was worth more than the rest of the auto industry combined was always bubble insanity, and it looks like the Tesla bubble is finally bursting. And this, of course, is why Musk is grabbing on to AI and why he proposed OpenAI merge into Tesla: AI is the current marker for a stock to end up priced based on an imaginary future rather than its current performance. Musk needs to inflate Tesla again, and just being an EV maker won’t now do that.
4. This is fine
I'm almost boring myself now whenever I post anything about the era of mass search traffic for publishers drawing to a close. But then someone comes up with a new piece of researching showing an impact of between 25-60% traffic loss because of Google's forthcoming Search Generative Experience. The fact that Google effectively does not allow publishers to opt out of SGE — you have to opt out of Googlebot completely to do so — should be an indication that Google has no intention of following the likes of OpenAI in paying to license publisher content, too. And I think the SGE is just the first part of a one-two punch to publisher guts: computers and how we access information is going to become more conversational and less focused on searching and going to web pages. As that happens, the entire industry will change, and it could happen faster than we think.
5. Feudal security
I often link to Cory Doctorow's posts, and it's not just because he's a friend -- it's because a lot of the things that he's been talking about for years are beginning to be a lot more obvious, even to stick-in-the-muds like me. This piece starts with a concept that I have struggled to articulate -- feudal security — and sprints on from there.
6. LLMs are terrible writers
Will Pooley has written a terrific piece from the perspective of an academic on why LLMs just don't write in a way which sounds human. They don't interrogate or question terms (because they have no concepts, so can't), there is no individual voice, they make no daring or original claims based on the evidence, and much more. My particular favourite — and one I have encountered a lot — is that LLMs love heavy sectionisation and simple adore bullet points. I've got LLMs to write stuff before, specifically telling them not to use bullet points, and they have used them anyway. As Tim succinctly put it in a post on Bluesky, LLMs create content which is “uniformly terrible, and terribly uniform”.
7. Craig Wright is not Satoshi Nakamoto
Craig Wright spent a lot of time claiming he was the pseudonymous creator of Bitcoin, and suing people on that basis. Finally, a court has ruled that he was lying. Whoever Nakamoto is/was, he's probably on an island somewhere drinking a piña colada.
8. Google updates, manually hits AI-generated sites
You might have noticed that Google did a big update in early March, finally responding to what everyone had been saying — that search had become dominated by rubbish for many search terms. Smarter people than me are still analysing the impact of that update, but one thing which stood out for me is there was a big chunk of manual actions to start. Manual actions are, as the name suggests, based on human review of a site, which means they are a kind of fallback when the algorithm isn't getting it right. And guess what the manual actions mostly targeted? AI content spam. All the sites that were whacked had some AI-generated content, and half of them were 90-100% created by AI. Of course, manual action is not a sustainable strategy to combat AI grey goo, but it should be a reminder to publishers that high levels of AI-generated content are not the promised land of cheap good content without those pesky writers. If you want to use it, do it properly.
9. The web is 35 years old, and Tim Berners-Lee is not thrilled
The web was meant to be a decentralised system. Instead, it's led to the kind of concentration of power and control that would have made the oligarchs of the past blush. That's just the starting point of Tim Berners-Lee's article marking the web's 35th anniversary, and he goes on to provide many good suggestions. I don't know if they are radical enough — but they are in the right direction.
10. A big tech diet
It's a long-standing journalistic cliché to try some kind of fad diet for a short period of time and write up the (usually hilarious) results, but in this "diet" Shubham Agarwal tried to drop products from big tech companies, and of course, it proved harder than you would think. Some things are pretty easy — swapping Gmail for Proton isn't hard (and Shubham missed out some tricks, like using forwards to redirect mail). But it's really difficult to avoid some products, like WhatsApp or LinkedIn, because there are few/no viable alternatives. That, of course, is just how the big tech companies like it because they long-ago gave up on the Steve Jobs mantra of making great products that people wanted to buy in favour of making mediocre products that people have no alternative to using.
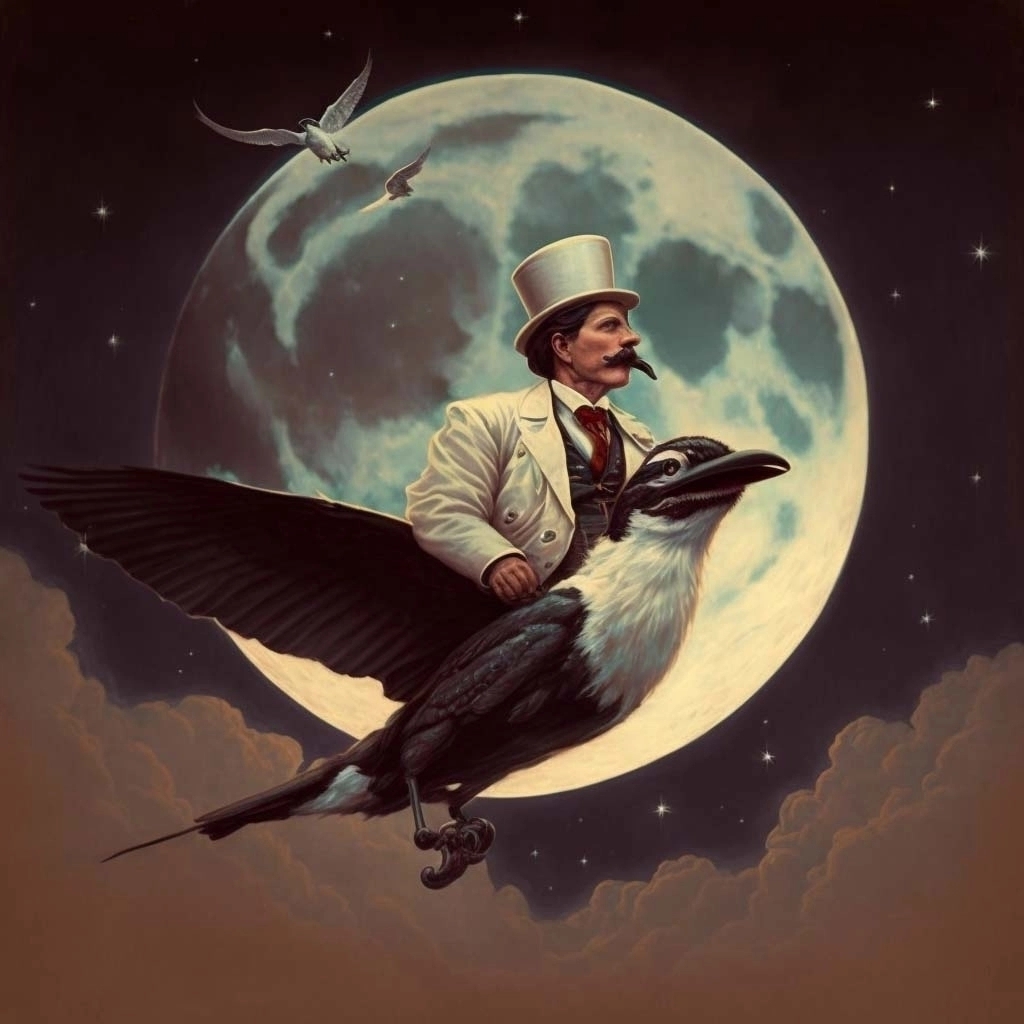
The end of the line for Google
“Personally, I don’t want the perception in a few years to be, ‘Those old school web ranking types just got steamrolled and somehow never saw it comin’…’”
Google engineer Eric Lehman, from an internal email in 2018, titled “AI is a serious risk to our business”
I should, of course, have put a question mark at the end of the title of this, but I very much do not want to fall foul of my own law. And, of course, talking about the end of the line for Google as a company is like talking about “the end of the line for IBM” in 2000, or “the end of the line for Microsoft” in 2008. Whatever happens, Google has so much impetus behind it, so much revenue, that a quick collapse is about as likely as my beloved Derby County winning League One, Championship and Premier League in three consecutive years. It isn’t happening, much as I might dream.
This is one of the reasons I quipped that Google could see the $2.3billion that Axel Springer and other European media groups want for its alleged monopolisation of digital advertising as “just the cost of doing business.” It’s the equivalent of someone having to pay a £250 fine for speeding: annoying, but not the end of the world, and not actually that likely to keep you down to under 70mph in the future.
Google’s problems, though, do run deep. Other than, as my friend Cory Doctorow has noted, the 1.5 good products it invented itself (“a search engine and a Hotmail clone”), the most successful Google products are acquisitions. Android? Acquired. YouTube? Acquired? Adtech? Acquired. Even Chrome, which dominates web browsing in a way which many people (including me) find more than a little scary, was based on Apple’s WebKit rendering engine – which was, in turn, based on the open source KHTML.
The fact is, Google is incredibly bad at successfully bringing products to market, to such a degree that no one trusts them to do it and stick with it for long. It continually enters markets with fanfare, only to exit not long after.
Take social networking. You probably remember Google+ (2011–2019). You may even remember Orkut (2004–2014). Perhaps you know about Google Buzz (2010–2011). But do you remember Jaiku, an early Twitter competitor which Google bought – and buried? The resources of Google could have been used to accelerate Jaiku’s development and – perhaps – win the battle against Twitter and the nascent Facebook. Instead, the company took two years rebuilding Jaiku on top of Google’s App Engine, with no new features or marketing spend to support the product. Two years later, they killed it.
What Google is pretty good at is producing research. Its 2017 paper on transformers directly led to many of the large language model breakthroughs which OpenAI used to create ChatGPT. Failing to spot the potential for its research isn’t unknown in technology history, but really great companies don’t allow others to turn themselves into competitors worth $80 billion on the back of it.
And particularly not when those other companies create technology which directly threatens core businesses, in this case, Google’s “one good product” – search. The bad news for Google is that even in the middle of last year, research showed people using ChatGPT for search tasks performed just as well as using a traditional search engine, with one exception — fact checking tasks. That, of course, is a big exception, but ordinary people use search engines for a lot more than just checking facts.
What’s also notable about the same research is that ChatGPT levelled the playing field between different educational levels, giving better access to information for those who have lower educational achievement. That strikes at the heart of Google’s mission statement, which promotes its goal of “organis[ing] the world’s information and making it universally accessible and useful” (my italics). Search, as good as it is, has always required the user to adapt to it. Conversational interaction models, which ChatGPT follows (the clue is in the name), change that profoundly.
In The Innovator’s Dilemma, Clayton Christiansen talks about the difficulties that successful companies have in sustaining innovation. Established businesses, he notes, are excellent at optimising their existing products and processes to serve current customers (this is called “sustaining innovation”). However, they often struggle when faced with a “disruptive innovation” – a new technology or business model that creates a whole new market and customer segment.
One of the potential solutions to this which Christiansen looks at is structural: Creating smaller, independent units or spin-offs tasked with exploring the disruptive technology can allow them to operate outside the constraints of the main company. This, of course, is probably what Google intended to do when it changed its structure to create Alphabet, a group of companies of which Google itself is just one part.
The biggest problem with this putative solution is that if you do it well, innovation doesn’t necessarily flow to where it is most needed. Google’s search products needed to seize on the research made in 2017 and integrate it. It didn’t, and – worse still – no one saw this as a potential disruption of the core business. The blinkers were too firmly on.
Perhaps that’s changing. Notably, last year that Google moved all its AI efforts into a single group, Google DeepMind. The “Google” in its name is significant: previously DeepMind was a separate business within Alphabet (and, in true Google style, it was acquired rather than built in-house). Now, on the surface, it looks likely to focus more on continuing Google’s mission, which means disrupting the traditional ten blue links.
Can it succeed? I’m not optimistic (publishers, take note). What we have here is a company which is good at research, but not at exploiting it; whose history is of one good product and a good Hotmail clone; that has a terrible record of announcing, releasing, and killing products, often multiple efforts in different categories all of which fail; and which has failed to keep its core product – search – up to date.
Perhaps the real question isn’t whether Google has reached the end of the line, but how exactly it made it this far?
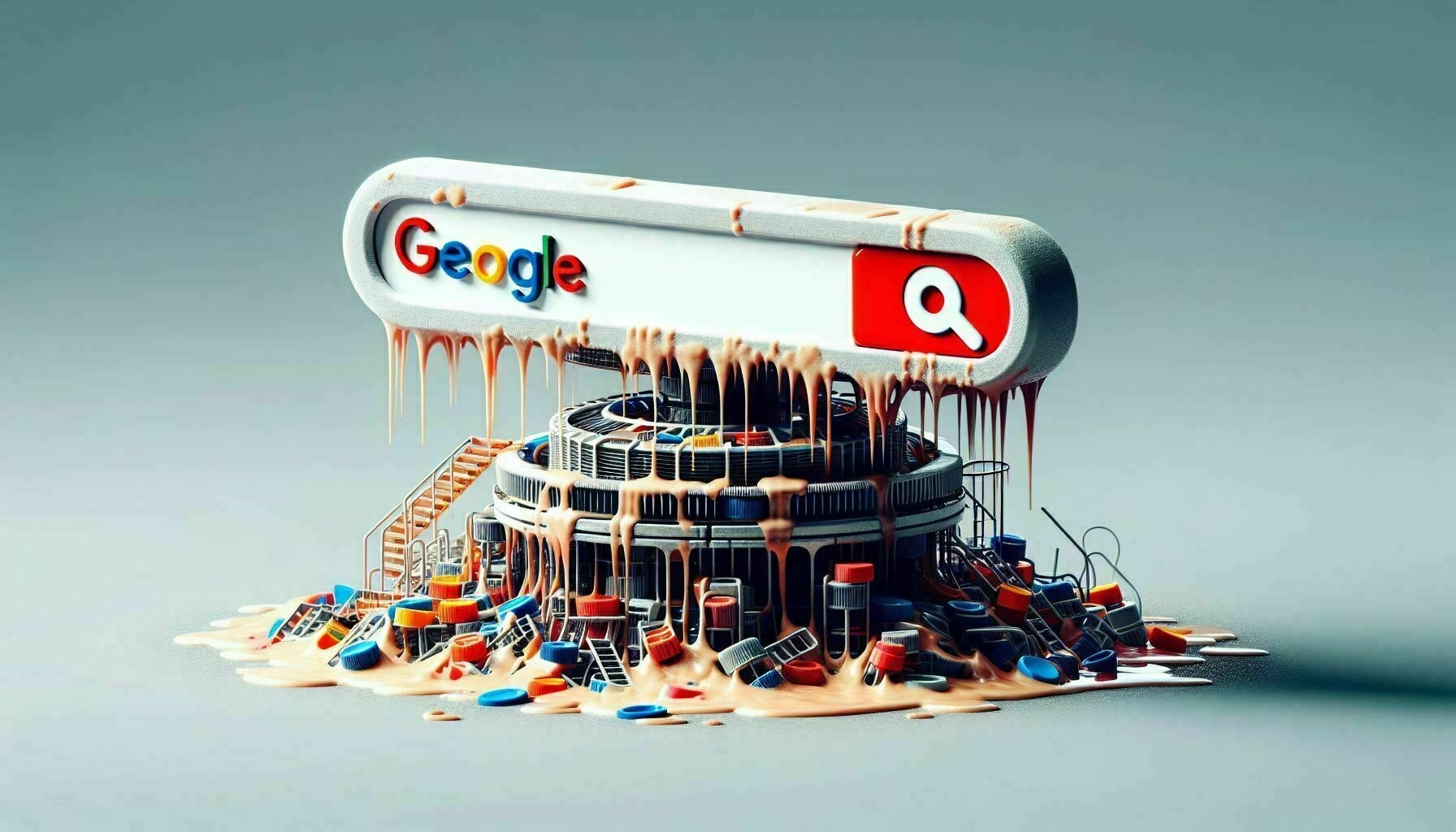
The information grey goo
I’m broadly positive about the future of LLMs and AI, but no one should pretend there will not be difficulties or that the transition to using machines isn’t going to pose plenty of challenges.
Some scenarios, though, are profoundly dangerous, not just for the publishing and creative industries, but for society as a whole.
When we discuss the threat of AI, many people imagine rampant machine intelligences with big guns hunting us all down in a post-apocalyptic wasteland (thank you, James Cameron). I doubt that’s likely. But one consequence which I can see use sleepwalking into is the informational equivalent of an apocalypse that dates back over thirty years: the “grey goo” scenario.
“Grey goo” was a concept which emerged when nanotechnology was the hot new thing. First put forward by Eric Drexler in his 1986 book The Engines of Creation, this is the idea that self-replicating nanobots could go out of control and consume all the resources on Earth, turning everything into a grey mass of nanomachines.
Few people worry about a nanotech apocalypse now, but arguably we should be worried about AI having a very similar effect on the internet.
Nowhere is safe
Unless you haven’t been paying attention, you will have noticed that the amount of content created by LLMs has been increasing at a vast rate. No one knows how much content is being generated, but SEOs – whose job it is to understand content on the internet – are concerned. Less ethical SEOs have used a combination of scraping and generative AI to quickly create low-quality sites with tens of thousands of pages on them, reaping rewards in traffic from Google over the short term.
The problem for Google is that creating a site like that is the work of perhaps a week – and probably a lot less if it can be automated – while it takes months for the search engine to spot that it’s a low-quality site. With more automated approaches, it will become trivial to create spammy sites far faster than Google can combat them. It’s like a game of whack-a-mole, where there are moles appearing at an exponential rate.
And Google isn’t the only platform which AI is threatening to turn to mush. Amazon has a issue with fake reviews generated by AI. And although it claims it is working on solutions, it appears to be incapable of even spotting fake AI-generated product names.
But what about human-to-human social networks? They have already been flooded with AI-generated responses. And it will only get worse, as companies create tools which let brands automatically respond to posts based on keywords using AI-generated text. Sooner or later, saying something which suggests you are in the market for a new car will get you spammed by responses from Ford, Skoda, VW, Tesla, every car dealer in your area, every private second hand seller… you get the picture. Good luck trying to find the real people.
It is obvious that anywhere content can be created will ultimately be flooded with AI-generated words and pictures. And the pace of this could accelerate over the coming years, as the tools to use LLMs programmatically become more complex.
For example, think about reviews on Amazon. It will be possible to create a programme which says “Find all my products on Amazon. Where the product rating drops below 5, add unique AI-generated reviews until the rating reaches 5 again. Continue monitoring this and adding reviews.”
We are already at the point where you can use natural language to create specialist GPTs. The ability to create these kinds of programmes is ultimately going to in the hands of everyone. And this applies to every rating system, all surveys, all polls, all user reviews – and similar approaches can be created for any kind of content.
Can Google, Amazon and the rest fight back? Yes – but at great cost. And it’s not clear that even the likes of Google has the resources to effectively fight millions of users of AI creating billions of low-quality pages at an accelerating scale.
Model collapse
A side-by-side comparison of content created from the same prompt in ChatGPT 3 versus ChatGPT 4 Turbo will show you the difference. And humans are getting better at writing prompts and giving AI models the information they need to do a better job. So surely, this is just a short-term problem, and AI content will get “good enough” to not flood the internet with crap.
The issue is that there is a counterbalancing force at play. As more and more AI-generated content floods the public internet, more and more of that content will end up as training data for AI. Exacerbating this, quality publications are largely blocking AI bots, for entirely understandable reasons, which means less, and less higher-quality content is being used to train the next generation of models.
For example, researchers have noted that the LAION-5B dataset, used to train Stable Diffusion and many other models, already contains synthetic images created by earlier AI models. This is the equivalent of a child learning to draw solely by copying the images made by younger children – not a scenario which is likely to improve quality.
In fact, researchers already have a name for the inevitable bad outcome: “model collapse”. In this case, the content generated by AI’s stops improving, and starts to get worse.
The Information Grey Goo
This is the AI Grey Goo scenario: an internet choked with low-quality content, which never improves, where it is almost impossible to locate public reliable sources for information because the tools we have been able to rely on in the past – Google, social media – can never keep up with the scale of new content being created. Where the volume of content created overwhelms human or algorithmic abilities to sift through it quickly and find high-quality stuff.
The social and political consequences of this are huge. We have grown so used to information abundance, the greatest gift of the internet, that having that disrupted would be a major upheaval for the whole of society.
It would be a challenge for civic participation and democracy for citizens and activists, who would no longer be able to access online information, opinions, debates, or campaigns about social and political issues.
With reliable information locked behind paywalls, anyone unwilling or unable to pay will be faced with picking through a rubbish heap of disinformation, scams, and low-quality nonsense.
In 2022, talking about the retreat behind paywalls, Jeff Jarvis asked “when disinformation is free, how can we restrict quality information to the privileged who choose to afford it?” If the AI-driven information grey goo scenario comes to pass, things would be much, much worse.
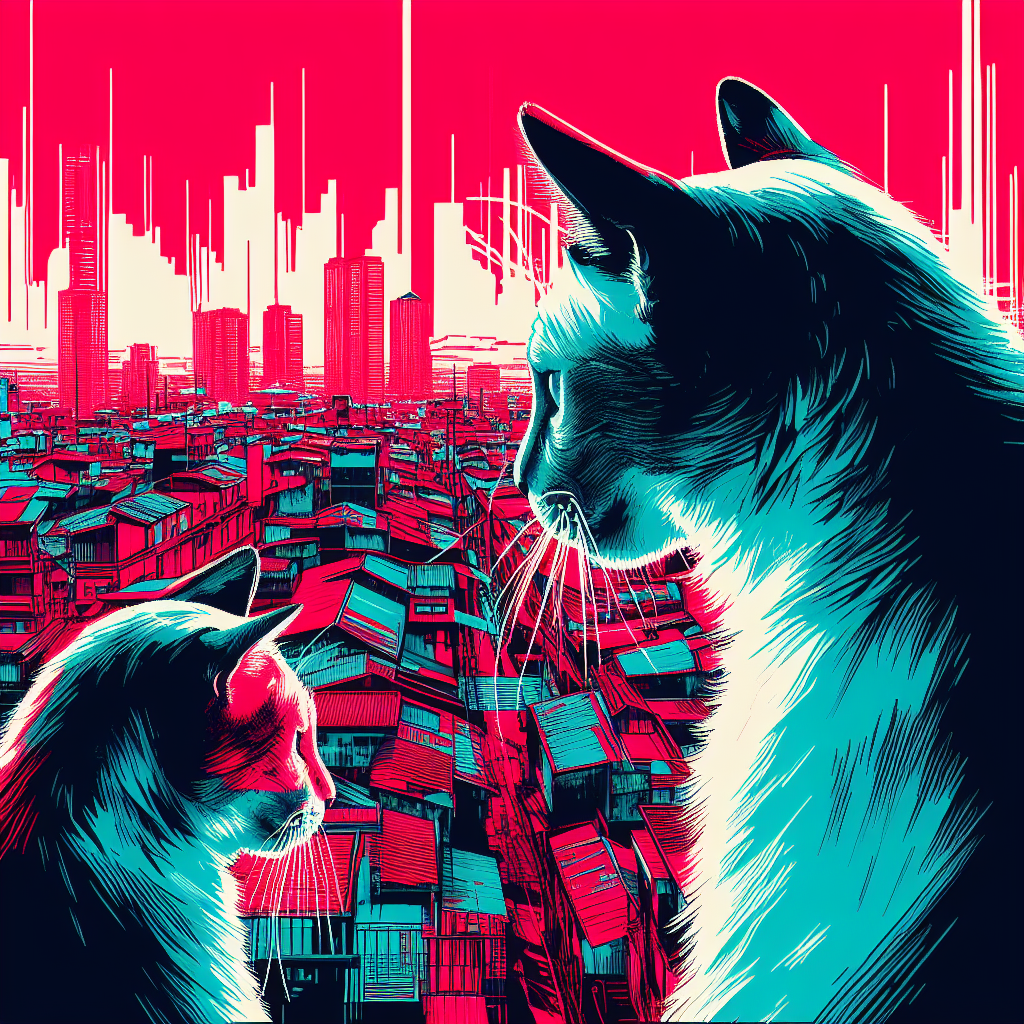
How to roll out AI in a creative business
I talked recently about how changing the culture of learning in your business will be important if you will make the most of AI. But no matter what, you’re going to have to roll it out – and you need to do that in a structured way.
Remember, this isn’t just an ordinary technology roll out: it’s a change management process that will have a lot of impact on your business. One framework which can help, and that I have found incredible powerful for managing change at scale is the ADKAR model of change management.
This model consists of five stages: Awareness, Desire, Knowledge, Ability, and Reinforcement. Each stage focuses on a different aspect of the change process, from creating a clear vision and generating buy-in, to acquiring the necessary skills and (importantly) sustaining the change over time, something that’s often neglected.
So how might you use ADKAR when looking at an AI rollout?
Awareness
At this point, your focus is to communicate the need and benefits of AI for your business, such as improving efficiency, enhancing customer service, or gaining insights. Explain how AI aligns with your vision, strategy and values, and what challenges it can help you overcome. Use data and evidence to support your case and address any concerns or misconceptions.
Remember, too, that this stage is about the need for change, not that change is happening. The most important outcome for this stage is that everyone understands the “why”.
Key elements of building awareness
- Start with your senior leaders. In effect, you need to go through a managed change process with them first, to ensure they are all aware of the need for change, have a desire to implement it, and have the knowledge they need to do so. Your senior team has probably been through quite a few changes, but none of them will have gone through what you are going to experience with AI.
- Explain the business drivers making the use of AI essential. Don’t sugar coat this, but be mindful of not using “doom” scenarios. Your model should be Bill Gates’ “Internet Tidal Wave” rather than Stephen Elop’s “Burning Platform”.
- For every single communication, ask yourself whether it contributes to helping employees be able to think "I understand why this change is needed". If not, rethink that comms.
- Be clear and consistent in messaging – and have leaders deliver the message (but make sure they are clear about it themselves).
- Tailor your message. Customize communication for different groups within the organisation. Different stakeholders may have different concerns and questions, so addressing them specifically can be more effective.
Desire
Building desire is all about cultivating willingness to support and engage with the change, and for AI, it’s incredibly important. While AI is a technology, it requires cultural change to succeed – and changing a company culture is very hard. Without building desire, any change which threatens the existing culture will fail.
There are many factors which influence whether you can create a desire for change. Personal circumstances will matter, and the fear with AI is that employees will lose their jobs. That’s a big barrier to building desire.
And, in some cases, those fears will not be misplaced, so it’s critical to be clear about your plans if you are to win enough trust to create desire. Consider, for example, making a commitment to reskill employees whose roles are affected by AI, rather than giving bland statements about avoiding redundancies “where possible”.
This is especially critical if you have a poor track record of managing change – so it’s vital that you are in touch with how your change management record really looks to your teams.
At this point, you should also identify your champions. Who, in the business, has a lot of influence? Who are the people who are at the centre of many things, who act as communicators? Who do other employees go to for help and advice? Are there people who, when a new project starts, are the first names on the list? They are not always senior, so make sure you’re looking across the board for your champions.
Even if they are not the most senior people or the most engaged with AI at this point, if you win them over and make them part of the project, you will reap the benefits.
Remember, too, that desire is personal to everyone. While making the business more efficient and profitable tends to get your senior team grinning, not everyone in your business is motivated by that. Focus, too, on the benefits for people’s careers, work/life balance, and especially with AI, freeing up time to do more creative things and less routine work.
And don’t, whatever you do, talk about how “if we don’t become more efficient, people will lose their jobs”. I’ve seen this approach taken many times, and in creative businesses, it almost never works. Desire is about motivating people to change, and fear is a bad motivator.
Key elements of building desire for AI:
- Inspire and engage your team members to participate in the AI adoption process.
- Identify and involve key influencers and champions who can advocate for AI and influence others.
- Highlight the personal and professional advantages of AI, such as learning new skills, increasing productivity, or advancing career opportunities.
- Create a sense of urgency and excitement around AI and its potential.
Knowledge
If awareness is about the why, the knowledge stage is about the how: how are we going to use these tools? This is where you build knowledge of the tools and the processes by which you use them.
One mistake that I have seen made – OK, to be honest, I have made – is to focus too heavily on training people on how to use a tool, without also training on changes in the processes you’re expecting people to make. Every new tool, including AI, comes with processes changes. And, in fact, the process changes that the tool enables are where you achieve the biggest benefits.
Training people in the context of the processes they follow (and any associated changes) relates the training to what people do – and that’s why I would recommend role-based training, which may cut across teams. If you have large teams, consider further segmenting this according to levels of experience. But I would recommend that you train everyone if possible: people who are left out may end up feeling either that AI isn’t relevant to them (and it will be) or that they have no future in your new, AI-enabled business.
Key elements of building knowledge of AI:
- Provide adequate and relevant training and resources for your team members to learn about AI and how to use it effectively. Make sure you document any process changes.
- Tailor the training to suit different learning styles, levels of expertise, and roles.
- Use a range of methods, such as workshops, webinars, online courses, or peer coaching.
- Encourage feedback and evaluation to measure progress and identify gaps.
Ability
So far, what we have done is all theory. This stage is where the rubber really hits the road because it’s where all that training starts to be implemented. And at this point, people will start to spot issues they didn't see before as they get the hang of new processes and get better at them. They will also find things you didn’t anticipate, and even better ways of using AI.
One aspect that’s critical at this stage is the generation of short-term wins. For a lot of your teams, AI is the proverbial big scary thing which is going to cost them their jobs – and even if you have had a successful “desire” phase, it can be easy for people to be knocked off course when that is at the back of their minds, or they are reading scare stories about how AI will mean the end of humanity.
Quick wins will help with this. They are positive, visible evidence about the success of people they know using AI, and in storytelling terms that is absolute gold dust. Remember, though, that the positives must be personal, and in a creative business they need to focus on improving the creative work. Shaving 10% of the time taken from a boring business process might be incredibly valuable to you, but it’s not all that compelling to a writer, editor, or video producer.
Key elements of building ability in AI:
- Support your team members to apply their AI knowledge and skills in their daily work.
- Create a safe and supportive environment where they can experiment, practice, and learn from mistakes.
- Provide guidance, feedback, and recognition to reinforce positive behaviours and outcomes.
- Make sure success stories are being shared, and that your teams are helping each other.
- Monitor and track performance and results to ensure quality and consistency.
Reinforcement
This stage focuses on activities that help make a change stick and prevent individuals from reverting to old habits or behaviours, and I think it’s both the most crucial stage of managing a change in technology or process – and the one that’s easily forgotten.
There are several reasons for this. First, commitment even among your senior team may be waning, leading to reduced encouragement from the top to continue along the path. The people who thought that your rollout of AI was likely to fail will probably be latching on to every bump in the road and turning them into roadblocks – ones that they “knew would happen”.
This is why it’s incredibly important to have all your senior team go through a parallel managed change process, to make sure they are all bought into what you want to achieve. AI is a strategic change on the same level of impact long-term as a complete restructure of your entire business, so there is no getting round managing that process for your senior team.
If you are starting to get resistance to AI deployment at this stage, check whether your senior team are still bought into it. In the worst case, some of them may be sending subconscious signals to their teams that they don’t have to keep going.
And now the bad news: in terms of budget, the reinforcement phase may cost as much as the training required in the knowledge phase because you need people looking after the AI roll out who are constantly engaging with your teams, understanding issues, celebrating success, and making sure that communications about how AI works for everyone, and – importantly – keeps everyone updated on new developments and changes.
For every new pitch, product or process, someone needs to be in the room asking how you can use AI to improve this, speed it up, or do interesting creative things. That is the only way they AI will become embedded in what you do, and not fade away – as so many corporate projects do.
Who is that person going to be? The likelihood is that in the “desire” phase, internal champions will emerge who can do that job. This offers the advantage of credibility, as it’s someone who is both personally familiar and professionally respected, but don’t make the mistake of assuming this role is something that you can tack on to a day job. Unless your business is very small, doing all this is a full-time role, for at least a year after you have “completed” the rollout of the technology.
Key elements of reinforcing AI use:
- Celebrate and reward your team members for their achievements and contributions to the AI adoption process.
- Focus on improvements in employees’ experience, not just business benefits.
- Solicit and act on feedback to improve and refine your AI practices and policies.
- Reinforce the benefits and value of AI for your business and your team.
- Keep your team informed and updated on the latest AI trends and developments and encourage continuous learning and improvement.
Weeknote, Sunday 10th December 2023
On Tuesday, I attended (virtually, of course) an International Association of News Media (INMA) talk given by Benedict Evans on the future of news. I like Ben’s approach, which is basically to keep reminding news people that putting all their eggs in the basked of Google and Facebook was a bad idea, and no amount of begging them for money is going to make it better.
As Cory often points out, the problem isn’t Google “stealing content” – it’s that Google and Facebook have an effective duopoly over online ads. They are stealing money rather than content. Focusing on MOAR COPYRIGHT isn’t going to fix that.
I spent far too much time this week futzing around with technology rather than doing anything productive with it. Tech is my absolute best (worst) prevarication method. Instead of just getting on and doing stuff with the tech, I spend time farting around with it, installing this, playing around with that. It gives me the illusion of doing something constructive when I’m actually doing nothing of the sort.
One thing I did was to change my contract with Ionos, which I use to host and hold the various domains I have. Back in the old days, I used to self-host WordPress, which I stopped doing when I managed to corrupt an entire database and lose about a decade’s worth of posts. I was still paying for services which I no longer needed, including legacy support for outdated versions of MySQL, so managed to cut down my costs quite considerably. I should have done that sooner.
One project which I might embark on is to trim back my online presences and consolidate into one site. I currently have my tech blog, this site, and also a Substack. Oh, and a small site on Writing.as for short fiction. I’m tempted to merge them all into one, on WordPress, which would be cheaper to run and potentially make more sense.
But I am definitely not embarking on this for a while. Too much other stuff to do.
One project which I really do have to get to grips with is consolidating all my files into a single, coherent place. Every time someone has launched a new online file storage system, I have tried it out. That used to be excusable – it was my job to know about stuff like this – but now it’s not, and it’s in desperate need of consolidation.
I have files on Dropbox, iCloud Drive, and two different OneDrives. There’s a lot of duplication, but the structure of all of them is quite different. It’s going to be a semi-manual mess to work out how to get it all in one place.
And that’s not even thinking about which place it should be. My main rule is that everything must be stored locally on at least one machine, which then gets locally backed up, and as the ThinkPad is the device with the most storage that rules out iCloud. OneDrive seems reluctant to store everything locally, even when I tell it to. That leaves Dropbox.
But that means paying for another storage service, which seems silly when I have a lot of OneDrive storage space. I have a personal Microsoft 365 account, for access to Word/Excel/PowerPoint, and that gives me 1Tb of OneDrive storage, effectively thrown in for nothing.
I have the free version of Dropbox, but because I have had it for a long time and they have done a lot of “get free storage” promos over the years, it gives me nearly 9Gb of free space. That’s enough for a decent-sized “working documents” folder, storage everything that’s in use. Everything else can be be archived easily.
So perhaps that’s a good first step: get everything on to the ThinkPad, which is easily done, sort all the files, and use Dropbox for “working documents”. Sounds like a plan.
But not for today.
Three things which caught my attention this week
- Timothy Burke is a journalist who had his devices seized by the FBI in an investigation into “leaked” Fox News footage. Having paid Google a lot of money over a long period for storage, he’s now been told Google is basically going to close his account and he has seven days to move hundreds of terabytes off their servers, or they will delete it. This is the danger of cloud storage: if you don’t have local copies, your files are not your own.
- Related, Cory wrote about how DRM allows companies to simply force you to accept downgrades to service. Which a bunch of PlayStation users found out the hard way when Sony simply removed content they had bought and paid for.
- Steven Levy wrote about Google’s NotebookLM, which is a service that lets you upload content and use a large language model to query it. This is a smart application of AI, but I want this to be local: I don’t want to have to upload my content into someone else’s servers.
This week I have been reading
Michael Jecks, thriller writer and pen expert (no really) has a new book out – the first that he’s self-published. It’s called One Last Dance Before I Die and as with all Mike’s books, it’s a well-constructed pacey read, which I would highly recommend if you want something fun and light.
I’ve also been reading Richard Skinner’s Writing a Novel, which is pretty good even if he is a bit snotty about genre fiction.
This week I have been writing
Remarkably little because I have been futzing around too much with technology. I did, though, write something last Sunday about resurrecting my MacBook Pro. The only downside I have found to that machine is that, compared to everything else I use, its keyboard really does suck. I’m so glad that Apple went back to proper switches.